In April 2020, the White House projected 200,000 deaths from Covid-19 even if ‘things are done almost perfectly” . The estimates in April by the White House were based on combining mean projections from leading epidemiological models from around the world as noted in an earlier post but given that deaths have gone beyond 230,000 as of Oct 27th either ‘things were not done perfectly’ or the estimates were off for these models and this post is examining the latter i.e. predictive ability of the Covid-19 models.
The chart below shows the differences between the mean estimates from 4 prominent epidemiological models and the actual confirmed cases in the US. The estimates from all of the 4 models were higher in March/April by multiple orders of magnitude and became slightly lower in July when the cases peaked in US and while the error range is narrowing for the models all of the models are still over-predicting the number of confirmed cases. The four models mentioned in the graph are Imperial College London (ICL) model ; the Institute for Health Metrics and Evaluation (IHME) model; the Youyang Gu (YYG) model and the London School of Hygiene & Tropical Medicine (LSHTM) model. To understand the divergence of the model projections and the confirmed cases, would first require an understanding the salient features of SEIR modelling which is available at the YYG site; two key adjustable model parameters are the reproductive number (Ro) which determines how many people are infected with one person having COVID-19 and the infection fatality rate (IFR) which estimates the percent of people infected with a disease who die from it and how these are estimated (from which test data) has an important role in the projections.
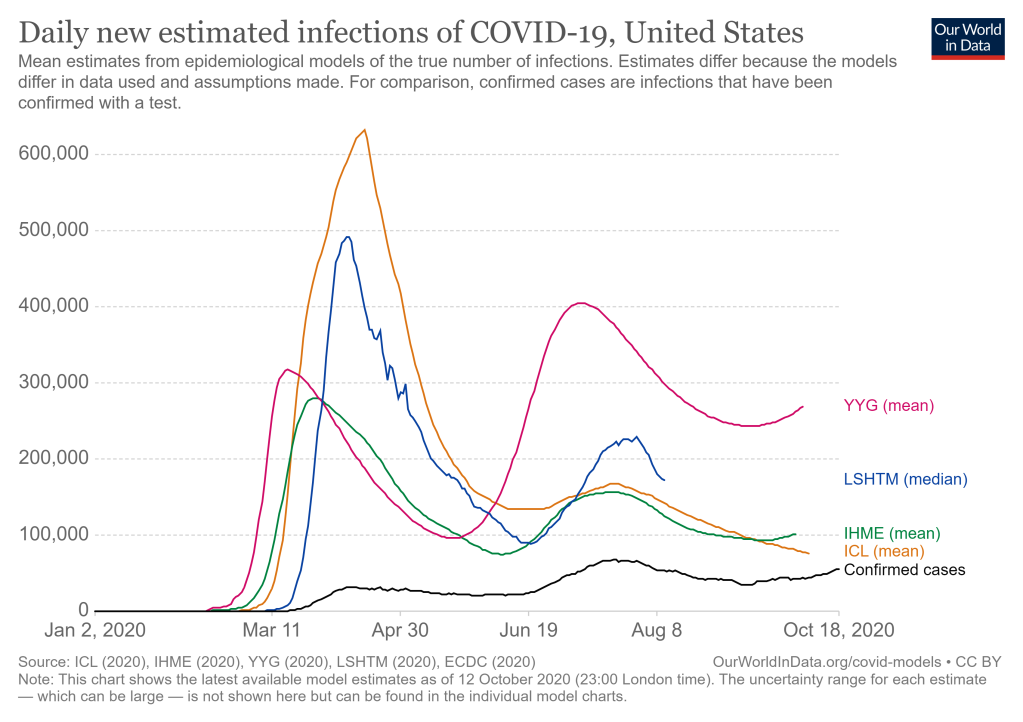
Two of the models whose mean projections are now tracking actual confirmed cases more closely as seen in above graph above are the ICL model and IHME model and I had examined them both in an earlier post. This post is examining updates and changes made to these two models since April which are have brought down the errors between their mean estimates and actual confirmed cases since April.
ICL Model
As background, the ICL model is a stochastic SEIR model and is a variation of the SEIR model since it adjusts the model parameters for age groups and accounts for 4 different infectious states as shown below rather than a single infectious state as in the traditional SEIR model. The IFR parameter is tweaked for each country based on different demography, social contact patterns and health systems in each country and the “average” IFR in the model ranges from 0.5% through to 1.5% depending on these factors.
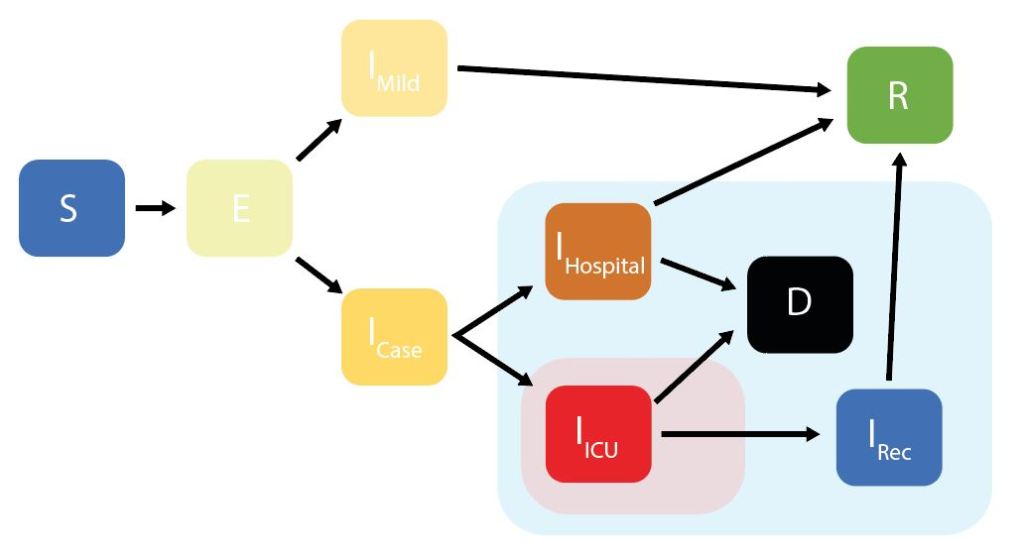
ICL Model Updates: The model parameters are updated 2-3 times weekly as per their website. The model is “fit” to data on confirmed deaths by using the estimated IFR to calculate how many infections would have been likely occurred over the previous weeks to produce the actual number of deaths. The key model parameter, the IFR was initially based on data from China but since then has been adjusted based on data from UK; list of all model parameters and assumptions is available at their website. The ICL model has had 5 upgrades since March; in version 1 and 2 the model incorporated impact of government interventions and used mobility data from Google using certain assumptions e.g. reducing mobility changes will reduce contacts outside the household but excludes gatherings in parks and residential places. Version 3 added a new parameter to account for different mobility patterns after lockdown ends and version 4 and 5 have added two more model parameters to de-couple the virus transmission post lockdown due to changes in social distancing being adapted.
IHME Model
The IHME model has had major revisions; the initial version of the model was not built as a traditional SEIR model in the sense that it did not account for an infection period, transmission of disease or incubation period or other features of Covid-19 as the ICL model does. Instead in the initial version, the IHME model used curve fitting (statistical techniques) to fit the data observed in China and Europe to estimate and project deaths and infections in the US which led to some widely publicized criticisms of the model. In their April update , IHME mentioned that the initial IFR for the model was based on data pulled from South Korea (since it had very high levels of testing) and then the IFR was updated with pooled data from other regions and random pooling from the Diamond Princess cruise ship infection data which has been estimated at 0.6% (95% uncertainty interval).
IHME Model Updates: The IHME model has had two major revisions in May 2020 to add two new model components: a statistical “death model” component which produces death estimates which are then used to fit an additional SEIR model component. The model now has 3 parts; the two components mentioned earlier and a transmission model and how these components work is that the IFR estimates are applied to COVID-19 deaths estimates from the ‘death model’ to produce age-specific rates of infection. These COVID-19 infection estimates, with COVID-19 death estimates are then feed into the transmission component of the model to project estimates of new infection.
The IHME death model has more assumptions than the ICL model and projects virus transmission as a function of mobility, temperature, testing rates, and the proportion of populations that live in dense areas. In addition, the model makes several assumptions about the relationship between confirmed deaths, confirmed cases, and testing levels and now has added a feature of projecting for 3 different scenarios – current scenario, mandates easing and mandate for universal masks. Similar to ICL model, this SEIR model is also ‘fit’ based on number of confirmed deaths to the output of the death model by using an estimated IFR to back-calculate the true number of infections.
Study on performance of Covid-19 models
Now that we know at a high level what changes have occurred in these 2 models since March, it would be worth examining the predictive performance of these two and other Covid-19 models. While looking for such studies, I came across a paper by IHME team published in July 2020 which examined the performance of 7 global Covid-19 models including the ICL and the IHME models; the other models included in study were the YYG model, two other variations of IHME model; a model developed by MIT (Delphi) and a model developed by Los Alamos National Lab (LANL). The study focused on errors in forecasting cumulative deaths and used mean absolute percent error (MAPE) as a metric across all 7 models. The study concluded that;
- forecasting errors increase for all models as the horizon period for forecasting increases; the average median absolute percent error (MAPE) for all models increased from 2.3% at one week to 32.6% at ten weeks.
- the overestimation by the transmission models (exponential growth) does not take into account ‘behavioral responses of individuals and governments’ since both they are ‘modifying behaviors and imposing mandates’ to restrict transmission.’
- MAPE at six weeks was less than 20% for YYG, LANL, IHME and Delphi models. The ICL model had much higher errors than other models reaching 20 times the error in tenth week.
- lower errors are noted were High income countries and largest errors were noted for African countries showing gaps in reporting outside developed countries.
The study also makes a recommendation that policymakers should consider an ‘ensemble’ of Covid-19 models given the range of errors noted rather than relying on one model by policymakers and interestingly that the Center for Disease Control (CDC) in US has been doing just that and that will be examined in a future post.
The standard SEIR model and anything built on it comes with a hidden false assumption that the distribution of infectious times is exponential. On my site ‘Modeling the COVID-19 Epidemic at Home’ I discuss a fix for SEIR that enables it to model a discrete set of infectious times.
Do you know if the SEIR based models you mention have done anything to improve their modeling of infectious time?
LikeLike